

Multi-scale approach to measuring residential segregation and
the case of Yaffo, Tel-Aviv
Itzhak Benenson and Itzhak Omer
Department of Geography and Human Environment, Tel Aviv University,
Israel
bennya@post.tau.ac.il, omery@post.tau.ac.il
Abstract
An approach to the individual-based description of segregation phenomena
is proposed. The proposed framework is based on hierarchical representation
of urban residential space and the estimation and comparison of individual
spatial segregation at different levels of the hierarchy.
Keywords: residential distribution, local segregation measures, GIS,
population census
Introduction
The measurement of urban residential patterns provides a framework for
verifying theories and models of migration and residential choice (Benenson,
1999; Portugali, 2000). With only some exceptions (Waldorf, 1992; Schnell
and Benjamini 1999), the measures or segregation indices (Peach, 1975;
Massey and Denton, 1988) utilise aggregate data, namely, numbers or fractions
of groups over given partitions of the studied area. The resulting global
measure is a single number that represents the state of an average member
of a group over the entire area. Contrary to the "averaged" view, the segregation
state of particular individual depends on his/her location. A person can
exist simultaneously in strong segregation regarding the other residents
in his/her house and in weak segregation regarding the residents of neighbouring
houses. Consequently, the series of estimates representing the segregation
state of individual with respect to the residents within different neighbourhoods
should be constructed and applied at each specific location.
The inability of the group approach to account for spatial relations
between individuals was understood more than two decades ago (Lee, 1978,
Peach, 1979), although it could not be replaced until necessary geo-referenced
personal data were made available (Boal, 1987). The situation changed in
the mid 1990s, when population censuses in several European countries,
including Israel, began including for the first time, exact geo-referencing
of householders and households (ICBS, 2000). This detailed spatial data
allows the development of individual-based local measures of
segregation at the resolution of houses, streets, neighborhoods, and so
forth.
The aim of this paper is to suggest a framework for the individual-based
description of segregation phenomena. The proposed framework is based on
hierarchical representation of urban residential space and the estimation
and comparison of individual spatial segregation at different levels of
the hierarchy. Conceptually, our approach follows the humanistic-phenomenological
stream in human geography (Relph, 1976; Tuan, 1977; Sack, 1980), with its
stress on personal experience and relationships. We apply the proposed
approach in a study of residential patterns in the Yaffo region of Tel-Aviv.
The concept of spatial hierarchy
A human being can belong to and exist in several spatial aggregates concurrently
and needs "borders" or "edges" of the place he/she perceives at a moment
(Tuan, 1977; Johnston, 1991). The spatial aggregates the person perceives
are, usually, hierarchically organized. The hierarchy of an individual's
experience regarding the built environment can, in turn, be defined by
them (Relph, 1976; Ley, 1983). For example, the level of houses can serve
as the basis of a person's hierarchy; the "home area" (Lee, 1968), that
is, the population of the closest neighboring houses, can be considered
as the next aggregate; and so forth. We will compare two approaches to
the hierarchical representation of space, which we illustrate with an example
from the Yaffo residential area of Tel-Aviv.
Bottom-up approach
The units of the lowest level L0 of the hierarchy D
represent individuals' locations (apartments). The units of the upper levels
are defined recursively, according to the neighborhood relations between
the inhabitants of the units of L0. The choice of these
units depends on the researcher's objectives, although houses seem the
natural choice to serve as units of level L1. The units
of the next level L2 can be defined as sets of houses
neighboring to the L1 - house. The definition of neighboring
houses is of a principal value for constructing the bottom-up hierarchy,
later we propose an approach for obtaining that definition. Neighborhoods
of increasing dimensions can be considered as units of levels L3,
L4, L5, until the highest possible
level of the city as a whole, LC, is reached. For each
individual A, therefore, we consider the series of embedded spatial
units L0A, L1A,
… LCA as representing different levels of
a nested hierarchy with A as the center. It is worth noting that according
to the bottom-up hierarchy, each individual house belongs to several units
at each level beginning at the level L2, while defines
one of these units only.
Top-down approach
The administrative division of a city can be used to determine hierarchy
D as well. Usually, the city has up to five or six levels of administrative
divisions, defined by regions, electoral districts, boroughs, ZIP zones,
and so forth. Each unit of a higher level (e.g. region) is subdivided into
the units of a lower level (electoral district). At each level of administrative
hierarchy units do not intersect and an individual belongs to one of them
only.
Top-down and bottom-up hierarchies for the Yaffo residential area
The top-down hierarchy D
for Yaffo (Fig. 1) contains five levels: LC, the "city"
of Yaffo; L4, the statistical areas; L3,
electoral districts; L2, the street cells (defined below,
see the "Yaffo case" section); and, finally, L1, the
level of houses. According to administrative hierarchy the neighboring
houses to a houses H at level n are those located within
the unit of level n that contains house H. That is, all the houses
within the street cell comprise the neighborhood of each separate house
within this cell at the level of cells, and so on.
Regarding the bottom-up approach, the most important step is the transition
from the level L1 to L2, where we are
required have to define the immediate spatial proximate of each house H. |
|
To do so in an intuitively acceptable manner that avoids computational
complexities, we use the coverage of Voronoi polygons constructed around
the centroids of populated buildings (Fig. 2).
Two houses are considered as neighbors if their Voronoi polygons have
a common edge. Consequently, we define first order neighborhood of a house
H as a set of houses U1(H), where each G Î
U1(H) satisfies the following conditions:
1. G is adjacent to H;
2. The distance between the centroids of G and H is less
than a given threshold value (we set it equal to 100 m for the case of
Yaffo);
3. G and H are on the same side of a street if it has
two or more traffic lanes;
Higher-order neighborhoods are defined recursively. Namely, the neighborhood
Uk(H) of the order k (k = 2, 3, …) is defined
as Uk-1(H) plus houses next to houses of Uk-1(H):
Uk(H) = {F | F Î
U1(G) AND G Î
Uk-1(H)} (1)
Figure 3 presents the resulting hierarchy.
Indices of spatial segregation
Global indices
As noted above, one can find a dozen analytically different global segregation
indices used in geographic research as of the early 1950s (Geary, 1954;
Duncan and Duncan, 1955). The indices can be roughly classified according
to the phenomena they are meant to reveal - dissimilarity, exposure, clustering,
and so on (Massey and Denton, 1988). In parallel, the indices differ according
to the number of population groups they relate to. The indices of spatial
autocorrelation (used primarily for recognition of homogeneous spatial
clusters) relate to members of a single population group (Getis and Ord,
1996). Most other indices estimate relationships between two groups (Wong,
1997), while some indices - Moran joint count statistics for multi-colored
maps (Goodchild, 1986) or Kendall t
(Kendall, 1970) - operate with an arbitrary number of population groups.
Each global index represents the state of an average representative of
a given group with respect to a partition defined a priori. The most popular
is the dissimilarity index (Peach, 1975), while we consider the Geary index
of spatial heterogeneity (Goodchild, 1986) of the population distribution
according to characteristic f:
K1 = Sijwij||fj
– fi||/(s2SiSjwij)
(2),
where fi, fj are the values
of the characteristic at locations i and j; weights wij
define an a-priory "influence" of locations j on location i,
where Sijwij
= 1, and s2 denotes the variance of f.
Local indices
Geographical approaches to estimating local spatial segregation and local
measures of segregation phenomena were suggested during the 1980s (Getis
and Ord, 1992) and developed in parallel with geostatistics (see Cressie,
1993, for review). As a result, measures of spatial relationship applied
in geographical research share the title "spatial correlation/autocorrelation"
with those applied in geostatistics but differ from them formally. In this
paper we continue with a discussion of geographical measures of spatial
relationships and delay the comparison of geographical and geostatistical
measures to the future. Local indices of spatial segregation are applied
primarily to the examination of the spatial clustering of population groups.
Two of them, meant to reveal the homogeneous or heterogeneous domains over
the studied area, are considered below:
The index G of local autocorrelation proposed by P. Getis and K. Ord
(Getis and Ord, 1992, Getis and Ord, 1996), is based on a comparison of
the local average of a characteristic f to its global average. The G-version
ignores the value of characteristic f at the location itself and
accounts for its neighbors only, while the G*-version, which we use below,
accounts for the value of f at the location i itself:
G*i,n = R(SjÎ
Un(i)wijfj – <f>)/s
(3),
where <f> is a mean and s2 is a variance
of f, Sijwij
= 1 and R is a normalizing multiplier.
The global counterpart of Getis' indices is the average value of a
characteristic over the entire city. One can easily recognize the similarity
between G*i,n and a moving average over neighborhood.
It follows from (3) that positive and high values of G*i,n
are obtained when the mean value of f over a neighborhood is essentially
higher than the global mean and negative and relatively low when the local
mean falls below the global mean. Consequently, either high positive or
low negative G*i,n values identify relatively homogeneous
neighborhoods.
Local Geary indices K1 and K2 (Getis
and Ord, 1996; Anselin, 1995) estimate the variance of the characteristic
within the neighborhood.
K1i,n = R1SjÎ
Un(i)wij|fj – fi|/s
(4),
K2i,n = R2SjÎ
Un(i)wij(fj – fi)2/s2
(5),
where the terms have the same meaning as in (3).
The K1i,n and K2i,n are always positive;
the higher their value, the higher the heterogeneity of the neighborhood.
One can easily recognize the similarity between K2i,n
and the semivariance.
Local Getis indices correspond to the global average of the characteristic
f, while local Geary indices can be considered as components of the
global Geary segregation index (Anselin, 1995). Each global index can be
localized either formally, by expanding global segregation indices into
local components (Anselin 1995) or by simply reformulating the index's
analytical expression (Benenson and Omer, 2000). Here we do not consider
other local segregation indices, including the local Moran I (Anselin,
1995) and joint count statistics (Goodchild, 1986).
Statistical inference for local segregation indices
The problem of statistical inference for local segregation indices is rather
complex. Our goal here is to recognize the changes in population distribution
over space, while the standard geostatistic approach is based on the stationarity
of the distribution variance (Cressie, 1993, Pannatier, 1996). Two approaches
to statistical inference regarding local measures of segregation are considered
in the literature. The first one is based on maximum likelihood estimation
of the index variance and further normalizing of the index (Getis and Ord,
1996; Anselin, 1995). Such an approach is problematic unless the assumption
of stationarity, which we do not want to accept, is fulfilled. In addition,
maximum likelihood approach results in indices varying within non-standardized
intervals; thus, the comparison of the indices calculated for different
situations becomes problematic. The alternative is a resampling
computational approach, based on Monte Carlo simulations (Anselin, 1995).
Resampling seems better suited to the poorly defined statistical situations
we are studying, especially because its relatively heavy calculations are
of minor importance when using state-of-the-art hardware. The limitations
of the scope of this paper prevent further discussion of the problem of
statistical inference, which will be delayed to a subsequent paper.
Measuring segregation according to spatial hierarchy
Definition
Let us consider some local segregation index S. For an individual
B located at unit i Î L0
and characterized by the value fB of characteristic f,
we estimate the value of Si,n at location i regarding
level Ln of D in the following way. First, select
neighborhood Un(i) of i, which belongs to Ln,
and estimate the values of fA for each individual A
located within Un(i); second, calculate Si,n
based on fB and the set of fA. Below,
we consider the level L0 to consist either of families
or of houses and map the spatial distribution of Si,n
over units i of L0.
The case of Yaffo
What follows is an attempt to understand what might result from the individual-based
approach when geo-referenced personal data are available. The main source
of data for this study is the Israeli Census of Population and Housing
for 1995 (ICSB, 2000), which data are available for supervised study at
ICBS. Census data are geo-referenced at the personal level, that is, one
of the fields of the personal record contains a unique identifier of the
polygon representing the building a person lives in. Based on these data,
we can study the ethnic residential distribution in the Yaffo region of
Tel-Aviv, which is occupied by Arab and Jewish residents. The Yaffo area
is about 7 km2, and its population in 1995 was about
40,000. The Jewish majority comprised about 70% of the population and the
Arab minority the other 30%. Regarding ethnic distribution we based our
study on the householder identity, because mixed families are extremely
rare.
In addition to level of buildings, the census GIS of Tel-Aviv contains
layers of streets, statistical areas, electoral districts and open spaces
(ICSB, 2000). The ethnic residential distribution we investigate is based
on the "religion" of the person only, defined by the six categories of
the census questionnaire: "Jewish", "Moslem", "Druze", "Christian", "Foreign
worker", "unknown". For the purposes of the current study, we have combined
the categories of "Moslem", "Druze" and "Christian" into one entitled "Arab".
The "unknown" category is considered as "Jewish", because the majority
of these householders are new immigrants whose Jewish affiliation has yet
to be officially recognized. The top-down and bottom-up hierarchies D
of the Yaffo urban space are already presented above. Ethnicity is a binary
characteristic and to avoid inconveniences we skip the level of houses
and consider the level of houses as a basic level of D for the Yaffo
case.
We use MapInfo GIS with a Vertical Mapper add-in as tools for the geographical
analysis and ArcCad topological tools for constructing street cells. The
procedures for constructing neighborhoods based on Voronoi polygons and
for calculating local segregation indices are developed as MapBasic extensions
of MapInfo. In the following, we consider G*i,n and K1i,n
indices, set weights wij = 1/(Nn - 1), where
Nn is a number of units in Un(i), and set
coefficients R equal to one. To map the results of analysis, we
always divide the interval of the index's variation into three classes,
corresponding to high, low and intermediate values of the index.
Ethnic residential segregation according to a top-down hierarchy
To represent G*i,n we map the fraction of Arab population
in a unit for each level of Yaffo's administrative hierarchy (Fig. 4).
According to (3), the difference between G*i,n, and this
fraction is a constant number. Going down the levels of hierarchy, one
can see that the maps at the levels of statistical areas, electoral districts
and street cells (Fig. 4b-d) do not add much to understanding residential
segregation in Yaffo created by house-level map (Fig. 4a). In contrast,
low-resolution maps provide an essentially biased representation of the
situation due to the arbitrarily location of the boundaries of the administrative
units and the high number of unpopulated buildings found in Yaffo. It is
obvious that the bias increases when the spatial units grow (Figs. 4b-d).
We do not present here maps of K1i,n, which are uninformative
for units above the house levels and proceed to the bottom-up hierarchy,
which begins with houses in any case. Let us note here that with the bottom-up
approach, the mean size of a neighborhood of order 4 corresponds to the
size of a street cell and the size of a neighborhood of order 8 corresponds
to an electoral district.
Ethnic residential segregation according to a bottom-up hierarchy
Here we compare spatial distributions of the indices G*i,n
and K1i,n constructed for the level of houses versus
neighborhoods of orders n = 1, 2, 4 and 8 (Figs. 5 - 6). Prior to
analyzing each index separately, let us point out two important differences
that stem from a comparison of Fig. 4 and Figs. 5 - 6. First, it is difficult
to locate the boundaries between homogeneous areas in Fig. 4, whereas the
locations of the boundaries remain constant in Figs. 5 - 6. On the maps
in Figs. 5 - 6, constructed for higher levels of the hierarchy the boundaries
may "disappear", but they do not actually move in space. Second, the boundaries
between homogeneous/heterogeneous domains in Figs. 5 - 6 do not correspond
to the boundaries between street cells, electoral districts or statistical
areas, which define the administrative partition and the top-down hierarchy.
Local Getis G*
The impression we receive from the mapping of the areas of concentration
of the Arab and Jewish population in Yaffo, as seen in Fig. 5, is unambiguous.
We can clearly distinguish domains of homogeneous populations of Arabs
or of Jews; these domains are characterized by values of G*i,n
that are close to the maximum or the minimum for all n. The rest of the
Yaffo area, where for some or for all n the values of G*i,n
are far from the extremes found, cannot be uniquely classified because
the moderate values of Getis local index can be obtained in two ways. First,
they may result from relatively homogeneous Un(i), where
the fractions of the Arab population in all the houses are similar and
close to global fraction of Arabs in Yaffo. Second, they can be obtained
for heterogeneous Un(i), where the average fraction of
Arabs is close there global fraction in Yaffo. The houses for which G*i,n
is far from the extremes for all or some n comprise about 20% of the populated
houses in Yaffo. In order to understand the segregation situation of the
individuals inhabiting these houses, we continue the analysis by means
of the local Geary K1 index.
Local Geary K1
According to (4), K1i,n is low when the values of the
characteristic are similar for all the houses within Un(i)
(Fig. 6). Thus, in addition to homogeneous areas of extreme fractions of
Arabs and Jews disclosed by extreme values of G*i,n,
low values of K1i,n mark the locations where the ethnic
content of the houses is similar, although it is far from the extremes
of 1:0 or 0:1. To test for whether such locations exist, let us consider
the relationship between the two indices (Fig. 7). It is clear from Fig.
7 that in Yaffo's mixed neighbourhoods (those having a high percentage
of both Arabs and Jews) are always heterogeneous, that is, they contain
houses having all possible ratios of Arab to Jewish populations including
purely Jewish and purely Arab ones.
Description of personal segregation situations by means of local segregation
indices
Up to this point, we have reviewed the ability of indices to indicate domains
of homogeneous and heterogeneous ethnic structure. Our basic goal, however,
is to estimate the segregation situation of a single person who, during
the daily activity cycle, experiences all the neighborhoods simultaneously.
Let us consider a hypothetical member A of an Arab ethnic group
located in house i and then describe "the segregation situation of A
" in terms of the values of G*i,n and K1i,n
for different n.
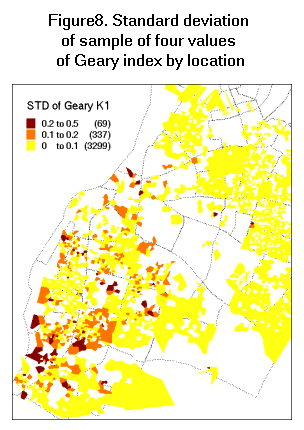 |
The simplest situation is that of individual A residing in house i,
which is located within a domain having the highest or lowest values of
G*i,n and close to zero K1i,n for all
n. Such an individual is located within an almost homogeneous Arab environment
within the house, at the level of adjacent houses and above, up to the
level of everyday local activity containing local shops and religious and
cultural centers - a neighborhood of order 8. High values of G*i,n
indicate that A is not exposed to the Jewish population until visiting
distant locations. When A is located in a house that is within an area
of minimal G*i,n (and, again, close to zero K1i,n)
for all n, the situation is reversed, that is, A is found within
a homogeneous Jewish environment within the house and at all levels up
to that of everyday activity.
The areas of either homogeneous Arab or Jewish population cover about
80% of Yaffo. Let us try to conceptualize the segregation situation if
A were located in a house in the remaining 20% of Yaffo's territory.
The basic properties of a person's segregation situation here are indicated
by K1i,n and its variation with n (Fig. 8). A
low K1i,n for all n characterizes a uniform situation,
one where A perceives each house in the neighborhoods Un(i)
as having equal and intermediate (due to low G*i,n) fractions
of an Arab population. The non-uniform case is characterized by K1i,n
fluctuating with n. Concerning Yaffo, non-uniform situations, that
is K1i,n varies with n are observed at locations within
the areas displaying close to zero values of G*i,n (Fig.
8). A's segregation state at such a locations depends on the range
of the fluctuations in K1i,n. If the range is high, then
A perceives all the possible variations of the ethnic structure
that can be observed in Yaffo within the radius of everyday local activity.
For the intermediate range of fluctuations, A remains within heterogeneous,
but predominantly Arab (characterized by high values of G*i,n)
or Jewish (low values of G*i,n) neighborhoods. |
Global estimates of Jewish-Arab segregation in Yaffo
The mean fraction of Arabs (corresponding to Getis G*i,n)
over the area we consider, which is bigger than the Yaffo administrative
region, equals 0.188. The global Geary index K1, calculated
for houses regarding neighborhoods of order 1 equals K1
= 0.099. Taken alone, these intermediate values demonstrate that the ethnic
residential distribution in Yaffo is not homogeneous, although its global
heterogeneity is not high. The local measures G*i,n and
K1i,n express this heterogeneity.
Conclusions
The purpose of this paper is to present the individual-based approach to
measuring residential segregation and to apply it to a real-world situation.
This approach enables the identification of areas of homogeneity according
to mean, variation and other characteristic of a residential distribution
and provides a multi-dimensional view of the segregation situation of a
person. A socio-geographic perspective on segregation demands further investigation
of the reasons for the observed phenomena. These reasons may be constraints
of the built environment, the tendency of similar householders to locate
in close proximity, and so forth. For example, in Yaffo the correlation
between the fraction of the Arab population and the architectural style
of a building is relatively high (Omer, 1996). Hence the latter can be
considered a factor contributing to the persistence of homogeneous Arab
or Jewish areas there.
A number of important issues await further study, including the problem
of statistical inference and the relation between standard methods of geostatistics
and local indices of segregation. From an applied point of view, however,
the most promising avenue seems the utilization of these indices for the
presentation of census data. Till now, the proposed partitioning of the
city into relatively homogeneous sub-areas had been based on uniting houses
according the mean values of the population characteristic there (Martin,
1998). To properly reflect the character of residential distribution we
have to account for its other properties, of which local variation seems
the most important.
References
1. Anselin L., 1995, Local Indicators of Spatial Association - LISA, Geographical
Analysis: 27 (2), 93 - 115.
2. Benenson I., 1999, Modeling Population Dynamics in the City: from
a Regional to a Multi-Agent Approach, "Discrete Dynamics in Nature and
Society", v3, 149-170.
3. Benenson, I, Omer I., 2000 Measuring Individual Segregation in Space
- A Formal Approach and Case Study. Submitted for publication.
4. Boal F.W., 1987, Segregation, In: M. Pasion (ed.), Social Geography:
Progress and Prospect, Croom Helm, Beckenham.
5. Cressie, N.A.C., 1993, Statistics for Spatial Data, Wiley &
Sons, NY, 900 pp.
6. Duncan O., Duncan B., 1955, A Methodological Analysis of Segregation
Indices, American Sociological Review, vol. 20, pp. 210- 217.
7. Geary, R.C., 1954, The Contiguity Ratio and Statistical Mapping,
Incorporated Statistician, v. 5, p. 115-141.
8. Getis A., Ord J.K., 1992, The Analysis of Spatial Association by
Use of Distance Statistics, Geographical Analysis, v24, p. 189-206.
9. Getis A., Ord J.K., 1996, Local Spatial Statistics: an Overview,
In P.Longley, M. Batty, Eds., Spatial Analysis: Modeling in a GIS Environment,
Cambridge (UK), Geoinformation International, 269 - 285.
10. Goodchild, M., 1986, Spatial Autocorrelation, CATMOG, 47, GeoBooks,
Norwich.
11. Israeli Central Bureau of Statistics, 2000, Socio-Economic Characteristics
of Population and Households in Localities and Statistical Areas. Pub.
No 8 in the 1995 Census of Population and Housing series, State of Israel,
Central Bureau of Statistics Publications, Jerusalem.
12. Johnston, R. J. 1991, People and Places in the Behavioral Environment.
In F. W. Boal and D.N. Livingstone (Eds) The Behavioral Environment, Routledge,
London.
13. Jones T.P, McEvoy D., 1978, Race and Space in Cloud-Cuckoo Lane,
Area 10, 162-166.
14. Kendall M.G., 1970, Rank Correlation Methods, 4th edition, London,
Griffen
15. Lambert J., Lambert C., 1982, Race, Ethnicity and Urban Change.
In A. Cochrane and L. McDowell, Urban Change and Conflict, Open University
Press.
16. Lee, T.R., 1968,Urban Neighborhoods as a Social-Spatial Scheme,
Human Relations, 21, 241-268.
17. Lee, T.R., 1978, Race, Space and Scale, Area, 10, 365-367.
18. Ley, D., 1983, A Social Geography of the City, Harper & Row,
New York.
19. Lieberson S., 1981, An Asymmetrical Approach to Segregation, In
C. Peach, V. Robinson, S. Smith (eds.), Ethnic Segregation in Cities, Croom
Helm, London.
20. Martin D., 1998 Optimizing census geography: the separation of
collection and output geographies. International Journal of GIS, v. 12,
579-606
21. Massey D., Denton N., 1988, The Dimensions of Residential Segregation,
Social Forces, vol. 76, pp. 281- 315.
22. Omer, I., 1996, Ethnic Residential Segregation as a Structuration
Process, Unpublished Ph.D Thesis, Tel-Aviv University, Tel-Aviv.
23. Pannatier, Y., VARIOWIN Software for Spatial Data Analysis in 2D,
Springer, New York, 1996, 91 pp.
24. Peach, C., 1975, Introduction: The Spatial Analysis of Ethnicity
and Class. In C. Peach (ed.), Urban Social Segregation, Longman, London.
25. Peach C., 1979, Race and Space, Area, 11, pp.82-89.
26. Portugali, J, 2000, Self-Organization and the City, Springer, Berlin,
352 pp.
27. Relph, E., 1976, Place and Placelessness, Pion, London.
28. Sack, R.D. 1980, Conceptions of Space in Social Thought: A Geographic
Perspective, Macmillan, London.
29. Schnell I., Benjamini Y., 1999, Socio-Spatial Lifestyles and Segregation,
www.cybergeo.presse.fr,
April, 1999.
30. Tuan, Y.F. 1977, Space and Place: The Perspective of Experience,
University of Minnesota Press, Minneapolis.
31. Waldorf B.S, 1993, Segregation in Urban Space: A New Measurement
Approach, Urban Studies, vol. 30 (7), 1151-1164.
32. Wong D., 1997, Spatial Dependency of Segregation Indices, Canadian
Geographer, 41 (2).